簡(jiǎn)單說明
分別使用OpenCV、ONNXRuntime部署YOLOV7目標(biāo)檢測(cè),一共包含12個(gè)onnx模型,依然是包含C++和Python兩個(gè)版本的程序。 編寫這套YOLOV7的程序,跟此前編寫的YOLOV6的程序,大部分源碼是相同的,區(qū)別僅僅在于圖片預(yù)處理的過程不一樣。YOLOV7的圖片預(yù)處理是BGR2RGB+不保持高寬比的resize+除以255 由于onnx文件太多,無法直接上傳到倉(cāng)庫(kù)里,需要從百度云盤下載,
YOLOV7的訓(xùn)練源碼是:
跟YOLOR是同一個(gè)作者的。
OpenCV+YOLOv7
推理過程跟之前的YOLO系列部署代碼可以大部分重用!這里就不在贅述了,詳細(xì)看源碼如下:輸出部分直接解析最后一個(gè)輸出層就好啦!
詳細(xì)實(shí)現(xiàn)代碼如下:
#include#include #include #include #include #include usingnamespacecv; usingnamespacednn; usingnamespacestd; structNet_config { floatconfThreshold;//Confidencethreshold floatnmsThreshold;//Non-maximumsuppressionthreshold stringmodelpath; }; classYOLOV7 { public: YOLOV7(Net_configconfig); voiddetect(Mat&frame); private: intinpWidth; intinpHeight; vector class_names; intnum_class; floatconfThreshold; floatnmsThreshold; Netnet; voiddrawPred(floatconf,intleft,inttop,intright,intbottom,Mat&frame,intclassid); }; YOLOV7::YOLOV7(Net_configconfig) { this->confThreshold=config.confThreshold; this->nmsThreshold=config.nmsThreshold; this->net=readNet(config.modelpath); ifstreamifs("coco.names"); stringline; while(getline(ifs,line))this->class_names.push_back(line); this->num_class=class_names.size(); size_tpos=config.modelpath.find("_"); intlen=config.modelpath.length()-6-pos; stringhxw=config.modelpath.substr(pos+1,len); pos=hxw.find("x"); stringh=hxw.substr(0,pos); len=hxw.length()-pos; stringw=hxw.substr(pos+1,len); this->inpHeight=stoi(h); this->inpWidth=stoi(w); } voidYOLOV7::drawPred(floatconf,intleft,inttop,intright,intbottom,Mat&frame,intclassid)//Drawthepredictedboundingbox { //Drawarectangledisplayingtheboundingbox rectangle(frame,Point(left,top),Point(right,bottom),Scalar(0,0,255),2); //Getthelabelfortheclassnameanditsconfidence stringlabel=format("%.2f",conf); label=this->class_names[classid]+":"+label; //Displaythelabelatthetopoftheboundingbox intbaseLine; SizelabelSize=getTextSize(label,FONT_HERSHEY_SIMPLEX,0.5,1,&baseLine); top=max(top,labelSize.height); //rectangle(frame,Point(left,top-int(1.5*labelSize.height)),Point(left+int(1.5*labelSize.width),top+baseLine),Scalar(0,255,0),FILLED); putText(frame,label,Point(left,top),FONT_HERSHEY_SIMPLEX,0.75,Scalar(0,255,0),1); } voidYOLOV7::detect(Mat&frame) { Matblob=blobFromImage(frame,1/255.0,Size(this->inpWidth,this->inpHeight),Scalar(0,0,0),true,false); this->net.setInput(blob); vector outs; this->net.forward(outs,this->net.getUnconnectedOutLayersNames()); intnum_proposal=outs[0].size[0]; intnout=outs[0].size[1]; if(outs[0].dims>2) { num_proposal=outs[0].size[1]; nout=outs[0].size[2]; outs[0]=outs[0].reshape(0,num_proposal); } /////generateproposals vector confidences; vector boxes; vector classIds; floatratioh=(float)frame.rows/this->inpHeight,ratiow=(float)frame.cols/this->inpWidth; intn=0,row_ind=0;///cx,cy,w,h,box_score,class_score float*pdata=(float*)outs[0].data; for(n=0;nthis->confThreshold) { Matscores=outs[0].row(row_ind).colRange(5,nout); PointclassIdPoint; doublemax_class_socre; //Getthevalueandlocationofthemaximumscore minMaxLoc(scores,0,&max_class_socre,0,&classIdPoint); max_class_socre*=box_score; if(max_class_socre>this->confThreshold) { constintclass_idx=classIdPoint.x; floatcx=pdata[0]*ratiow;///cx floatcy=pdata[1]*ratioh;///cy floatw=pdata[2]*ratiow;///w floath=pdata[3]*ratioh;///h intleft=int(cx-0.5*w); inttop=int(cy-0.5*h); confidences.push_back((float)max_class_socre); boxes.push_back(Rect(left,top,(int)(w),(int)(h))); classIds.push_back(class_idx); } } row_ind++; pdata+=nout; } //Performnonmaximumsuppressiontoeliminateredundantoverlappingboxeswith //lowerconfidences vector indices; dnn::NMSBoxes(boxes,confidences,this->confThreshold,this->nmsThreshold,indices); for(size_ti=0;idrawPred(confidences[idx],box.x,box.y, box.x+box.width,box.y+box.height,frame,classIds[idx]); } } intmain() { Net_configYOLOV7_nets={0.3,0.5,"models/yolov7_736x1280.onnx"};////choices=["models/yolov7_736x1280.onnx","models/yolov7-tiny_384x640.onnx","models/yolov7_480x640.onnx","models/yolov7_384x640.onnx","models/yolov7-tiny_256x480.onnx","models/yolov7-tiny_256x320.onnx","models/yolov7_256x320.onnx","models/yolov7-tiny_256x640.onnx","models/yolov7_256x640.onnx","models/yolov7-tiny_480x640.onnx","models/yolov7-tiny_736x1280.onnx","models/yolov7_256x480.onnx"] YOLOV7net(YOLOV7_nets); stringimgpath="images/dog.jpg"; Matsrcimg=imread(imgpath); net.detect(srcimg); staticconststringkWinName="DeeplearningobjectdetectioninOpenCV"; namedWindow(kWinName,WINDOW_NORMAL); imshow(kWinName,srcimg); waitKey(0); destroyAllWindows(); }
運(yùn)行測(cè)試如下:
審核編輯:劉清
-
OpenCV
+關(guān)注
關(guān)注
31文章
635瀏覽量
41594 -
python
+關(guān)注
關(guān)注
56文章
4811瀏覽量
85076
原文標(biāo)題:源碼 | OpenCV DNN + YOLOv7目標(biāo)檢測(cè)
文章出處:【微信號(hào):CVSCHOOL,微信公眾號(hào):OpenCV學(xué)堂】歡迎添加關(guān)注!文章轉(zhuǎn)載請(qǐng)注明出處。
發(fā)布評(píng)論請(qǐng)先 登錄
相關(guān)推薦
在英特爾AI開發(fā)板上用OpenVINO NNCF優(yōu)化YOLOv7
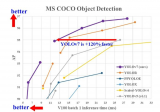
yolov7 onnx模型在NPU上太慢了怎么解決?
無法使用MYRIAD在OpenVINO trade中運(yùn)行YOLOv7自定義模型怎么解決?
深度解析YOLOv7的網(wǎng)絡(luò)結(jié)構(gòu)
YOLOv6在LabVIEW中的推理部署(含源碼)
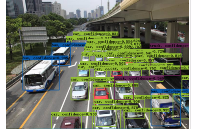
YOLOv7訓(xùn)練自己的數(shù)據(jù)集包括哪些
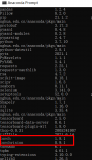
三種主流模型部署框架YOLOv8推理演示
yolov5和YOLOX正負(fù)樣本分配策略
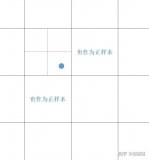
使用OpenVINO優(yōu)化并部署訓(xùn)練好的YOLOv7模型
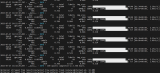
OpenCV4.8+YOLOv8對(duì)象檢測(cè)C++推理演示
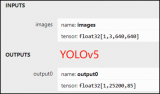
詳細(xì)解讀YOLOV7網(wǎng)絡(luò)架構(gòu)設(shè)計(jì)
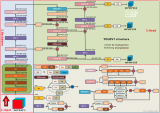
基于OpenCV DNN實(shí)現(xiàn)YOLOv8的模型部署與推理演示
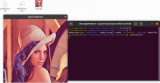
在樹莓派上部署YOLOv5進(jìn)行動(dòng)物目標(biāo)檢測(cè)的完整流程
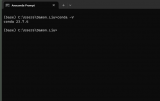
采用華為云 Flexus 云服務(wù)器 X 實(shí)例部署 YOLOv3 算法完成目標(biāo)檢測(cè)
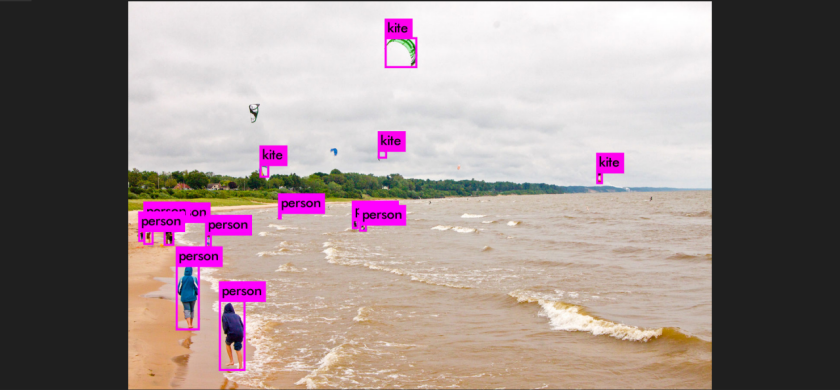
評(píng)論