10、OPTICS
OPTICS 聚類( OPTICS 短于訂購(gòu)點(diǎn)數(shù)以標(biāo)識(shí)聚類結(jié)構(gòu))是上述 DBSCAN 的修改版本。
我們?yōu)榫垲惙治鲆肓艘环N新的算法,它不會(huì)顯式地生成一個(gè)數(shù)據(jù)集的聚類;而是創(chuàng)建表示其基于密度的聚類結(jié)構(gòu)的數(shù)據(jù)庫(kù)的增強(qiáng)排序。此群集排序包含相當(dāng)于密度聚類的信息,該信息對(duì)應(yīng)于范圍廣泛的參數(shù)設(shè)置。
—源自:《OPTICS :排序點(diǎn)以標(biāo)識(shí)聚類結(jié)構(gòu)》,1999
它是通過(guò) OPTICS 類實(shí)現(xiàn)的,主要配置是“ eps ”和“ min _ samples ”超參數(shù)。下面列出了完整的示例。
# optics聚類
from numpy import unique
from numpy import where
from sklearn.datasets import make_classification
from sklearn.cluster import OPTICS
from matplotlib import pyplot
# 定義數(shù)據(jù)集
X, _ = make_classification(n_samples=1000,
n_features=2,
n_informative=2,
n_redundant=0,
n_clusters_per_class=1,
random_state=4)
# 定義模型
model = OPTICS(eps=0.8, min_samples=10)
# 模型擬合與聚類預(yù)測(cè)
yhat = model.fit_predict(X)
# 檢索唯一群集
clusters = unique(yhat)
# 為每個(gè)群集的樣本創(chuàng)建散點(diǎn)圖
for cluster in clusters:
# 獲取此群集的示例的行索引
row_ix = where(yhat == cluster)
# 創(chuàng)建這些樣本的散布
pyplot.scatter(X[row_ix, 0], X[row_ix, 1])
# 繪制散點(diǎn)圖
pyplot.show()
運(yùn)行該示例符合訓(xùn)練數(shù)據(jù)集上的模型,并預(yù)測(cè)數(shù)據(jù)集中每個(gè)示例的群集。然后創(chuàng)建一個(gè)散點(diǎn)圖,并由其指定的群集著色。在這種情況下,我無(wú)法在此數(shù)據(jù)集上獲得合理的結(jié)果。
圖:使用OPTICS聚類確定具有聚類的數(shù)據(jù)集的散點(diǎn)圖
11、光譜聚類
光譜聚類是一類通用的聚類方法,取自線性線性代數(shù)。
最近在許多領(lǐng)域出現(xiàn)的一個(gè)有希望的替代方案是使用聚類的光譜方法。這里,使用從點(diǎn)之間的距離導(dǎo)出的矩陣的頂部特征向量。
—源自:《關(guān)于光譜聚類:分析和算法》,2002年
它是通過(guò) Spectral 聚類類實(shí)現(xiàn)的,而主要的 Spectral 聚類是一個(gè)由聚類方法組成的通用類,取自線性線性代數(shù)。要優(yōu)化的是“ n _ clusters ”超參數(shù),用于指定數(shù)據(jù)中的估計(jì)群集數(shù)量。下面列出了完整的示例。
# spectral clustering
from numpy import unique
from numpy import where
from sklearn.datasets import make_classification
from sklearn.cluster import SpectralClustering
from matplotlib import pyplot
# 定義數(shù)據(jù)集
X, _ = make_classification(n_samples=1000,
n_features=2,
n_informative=2,
n_redundant=0,
n_clusters_per_class=1,
random_state=4)
# 定義模型
model = SpectralClustering(n_clusters=2)
# 模型擬合與聚類預(yù)測(cè)
yhat = model.fit_predict(X)
# 檢索唯一群集
clusters = unique(yhat)
# 為每個(gè)群集的樣本創(chuàng)建散點(diǎn)圖
for cluster in clusters:
# 獲取此群集的示例的行索引
row_ix = where(yhat == cluster)
# 創(chuàng)建這些樣本的散布
pyplot.scatter(X[row_ix, 0], X[row_ix, 1])
# 繪制散點(diǎn)圖
pyplot.show()
運(yùn)行該示例符合訓(xùn)練數(shù)據(jù)集上的模型,并預(yù)測(cè)數(shù)據(jù)集中每個(gè)示例的群集。然后創(chuàng)建一個(gè)散點(diǎn)圖,并由其指定的群集著色。
在這種情況下,找到了合理的集群。
圖:使用光譜聚類聚類識(shí)別出具有聚類的數(shù)據(jù)集的散點(diǎn)圖
12、高斯混合模型
高斯混合模型總結(jié)了一個(gè)多變量概率密度函數(shù),顧名思義就是混合了高斯概率分布。它是通過(guò) Gaussian Mixture 類實(shí)現(xiàn)的,要優(yōu)化的主要配置是“ n _ clusters ”超參數(shù),用于指定數(shù)據(jù)中估計(jì)的群集數(shù)量。下面列出了完整的示例。
# 高斯混合模型
from numpy import unique
from numpy import where
from sklearn.datasets import make_classification
from sklearn.mixture import GaussianMixture
from matplotlib import pyplot
# 定義數(shù)據(jù)集
X, _ = make_classification(n_samples=1000,
n_features=2,
n_informative=2,
n_redundant=0,
n_clusters_per_class=1,
random_state=4)
# 定義模型
model = GaussianMixture(n_components=2)
# 模型擬合
model.fit(X)
# 為每個(gè)示例分配一個(gè)集群
yhat = model.predict(X)
# 檢索唯一群集
clusters = unique(yhat)
# 為每個(gè)群集的樣本創(chuàng)建散點(diǎn)圖
for cluster in clusters:
# 獲取此群集的示例的行索引
row_ix = where(yhat == cluster)
# 創(chuàng)建這些樣本的散布
pyplot.scatter(X[row_ix, 0], X[row_ix, 1])
# 繪制散點(diǎn)圖
pyplot.show()
運(yùn)行該示例符合訓(xùn)練數(shù)據(jù)集上的模型,并預(yù)測(cè)數(shù)據(jù)集中每個(gè)示例的群集。然后創(chuàng)建一個(gè)散點(diǎn)圖,并由其指定的群集著色。在這種情況下,我們可以看到群集被完美地識(shí)別。這并不奇怪,因?yàn)閿?shù)據(jù)集是作為 Gaussian 的混合生成的。
圖:使用高斯混合聚類識(shí)別出具有聚類的數(shù)據(jù)集的散點(diǎn)圖
三、總結(jié)
在本教程中,您發(fā)現(xiàn)了如何在 python 中安裝和使用頂級(jí)聚類算法。具體來(lái)說(shuō),你學(xué)到了:
- 聚類是在特征空間輸入數(shù)據(jù)中發(fā)現(xiàn)自然組的無(wú)監(jiān)督問(wèn)題。
- 有許多不同的聚類算法,對(duì)于所有數(shù)據(jù)集沒(méi)有單一的最佳方法。
- 在 scikit-learn 機(jī)器學(xué)習(xí)庫(kù)的 Python 中如何實(shí)現(xiàn)、適合和使用10種頂級(jí)聚類算法
-
代碼
+關(guān)注
關(guān)注
30文章
4830瀏覽量
69095 -
數(shù)據(jù)分析
+關(guān)注
關(guān)注
2文章
1461瀏覽量
34176 -
python
+關(guān)注
關(guān)注
56文章
4809瀏覽量
85054
發(fā)布評(píng)論請(qǐng)先 登錄
相關(guān)推薦
一種基于聚類和競(jìng)爭(zhēng)克隆機(jī)制的多智能體免疫算法
一種改進(jìn)的BIRCH算法聚類方法
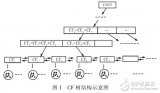
Python無(wú)監(jiān)督學(xué)習(xí)的幾種聚類算法包括K-Means聚類,分層聚類等詳細(xì)概述
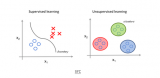
如何在python中安裝和使用頂級(jí)聚類算法?
一種自適應(yīng)的關(guān)聯(lián)融合聚類算法
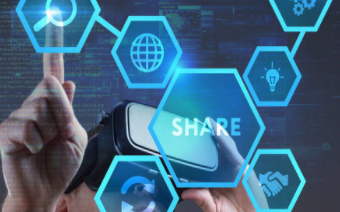
評(píng)論