EinSum介紹
EinSum(愛因斯坦求和)是一個(gè)功能強(qiáng)大的算子,能夠簡潔高效地表示出多維算子的乘累加過程,對(duì)使用者非常友好。
本質(zhì)上, EinSum是一個(gè)算子族,可以表示多種基礎(chǔ)操作,如矩陣乘法、Reduce。EinSum支持任意多的輸入,只要計(jì)算中只包含點(diǎn)乘(element-wise)、廣播(broadcast)、歸約求和(reduction sum)都可以使用EinSum來表示。以下給出一種將EinSum計(jì)算等價(jià)表達(dá)的流程:
- 將輸入的維度符號(hào)放入一個(gè)列表,移除重復(fù)元素后按升序排列;
- 對(duì)各輸入維度執(zhí)行轉(zhuǎn)置操作,確保維度標(biāo)識(shí)符按照升序?qū)R,實(shí)現(xiàn)維度對(duì)齊;
- 在缺失的維度上填充1(擴(kuò)展維度),以便與第一步中定義的維度保持一致;
- 對(duì)所有輸入執(zhí)行廣播點(diǎn)乘;
- 對(duì)那些不在輸出標(biāo)識(shí)符中的維度執(zhí)行累加操作;
- 利用轉(zhuǎn)置操作調(diào)整維度順序,使其與輸出標(biāo)識(shí)符的順序一致。
下圖是以out = EinSum("ijk, lki-> li", in0, in1)為例,根據(jù)上述步驟進(jìn)行等價(jià)轉(zhuǎn)換。
TPU-MLIR轉(zhuǎn)換
雖然使用上述流程可以完成對(duì)EinSum的計(jì)算轉(zhuǎn)換,但如果嚴(yán)格按照該流程執(zhí)行,會(huì)帶來大量的Transpose和Reshape操作,這不僅會(huì)給TPU-MLIR的LayerGroup功能帶來挑戰(zhàn),同時(shí)也難以顯式地識(shí)別出如矩陣乘法這類操作,從而無法充分利用硬件加速單元。因此,TPU-MLIR并未直接采用上述流程進(jìn)行轉(zhuǎn)換。
接下來,我們將詳細(xì)介紹EinSum的完整處理過程。
前端接口
以下示例代碼摘自O(shè)nnxConverter.py文件,并附帶了注釋。代碼整體結(jié)構(gòu)簡潔明了,我們可以看到,轉(zhuǎn)換函數(shù)目前僅支持兩個(gè)輸入的常見情況。特別需要注意的是公式的歸一化過程。由于EinSum的表達(dá)式可以使用任意非重復(fù)字符來表示下標(biāo),這雖然提高了可讀性,但也導(dǎo)致同一操作有多種不同的表示方式。歸一化操作就是將表達(dá)式字符重新映射,以字符'a'作為起始。例如,比如ij,jk->ik和dk,kv->dv都會(huì)映射為ab,bc->ac。
#https://pytorch.org/docs/1.13/generated/torch.einsum.html?highlight=einsum#torch.einsum
defconvert_einsum_op(self,onnx_node):
assert(onnx_node.op_type=="Einsum")
equation=onnx_node.attrs.get("equation").decode()
#公式歸一化
defnormalize_equation(equation_c):
equation=equation_c
new_equation=''
start='a'
translate_map={}
forsinequation:
ifs=='':
continue
elifnot((s>='a'ands<=?'z')or(s>='A'ands<=?'Z')):
translate_map[s]=s
elifsnotintranslate_map:
translate_map[s]=start
start=chr(ord(start)+1)
new_equation+=translate_map[s]
returnnew_equation
equation=normalize_equation(equation)
lhs=self.getOperand(onnx_node.inputs[0])#
#大多情況下rhs是Weight, self.getOp會(huì)先到Weight Map中查找;如果找不到,
#其會(huì)從Mutable Tensor中查找,然后返回對(duì)應(yīng)的Value。
rhs=self.getOp(onnx_node.inputs[1])
new_op=top.EinsumOp(self.unranked_type,
[lhs,rhs],
mode=StringAttr.get(equation),
#設(shè)置loc信息,方便找到原圖對(duì)應(yīng)算子
loc=self.get_loc("{}_{}".format(onnx_node.name,onnx_node.op_type)),
#將該算子插入到當(dāng)前的block中
ip=self.mlir.insert_point).output
#將輸出放到MutableTensor列表中,供后面算子使用
self.addOperand(onnx_node.name,new_op)
內(nèi)部轉(zhuǎn)換
TPU-MLIR目前支持了幾種常見的表達(dá)式,并根據(jù)不同的算子進(jìn)行了優(yōu)化轉(zhuǎn)換。所有的變換最終都利用了硬件的矩陣乘法加速單元,從而實(shí)現(xiàn)了對(duì)算子的有效加速。以下是部分代碼片段,該代碼來自tpu-mlir/lib/Dialect/Top/Canonicalize/Einsum.cpp,并在原有基礎(chǔ)上添加了注釋。
structConvertEinsum:publicOpRewritePattern{
usingOpRewritePattern::OpRewritePattern;
LogicalResultmatchAndRewrite(EinsumOpop,
PatternRewriter&rewriter)constoverride{
//目前只支持輸入個(gè)數(shù)為2或者輸入0為Weight的情況
if(op.getInputs().size()!=2||module::isWeight(op.getInputs()[0])){
llvm_unreachable("Notsupportnow.");
//returnfailure();
}
autonone=module::getNoneOp(op);
automode=op.getMode().str();
autolhs=op.getInputs()[0];
autorhs=op.getInputs()[1];
autolshape=module::getShape(lhs);
autorshape=module::getShape(rhs);
std::stringlname=module::getName(lhs).str();
std::stringrname=module::getName(rhs).str();
std::stringname=module::getName(op.getOutput()).str();
std::vectoroperands;
std::vectorattrs;
if(mode=="a,b->ab"){
//外積操作:可看作[a,1]x[1,b]的矩陣乘法操作
//lhs->ReshapeOp():shape=[a]toshape[a,1]
rewriter.setInsertionPointAfter(lhs.getDefiningOp());
//
autonewType=RankedTensorType::get({lshape[0],1},module::getElementType(lhs));
autoloc=NameLoc::get(rewriter.getStringAttr(lname+"_to2dim"));
autolrsOp=rewriter.create(loc,newType,ValueRange{lhs});
operands.push_back(lrsOp);
//rhs->ReshapeOp():shape=[b]toshape[1,b]
rewriter.setInsertionPointAfter(rhs.getDefiningOp());
newType=RankedTensorType::get({1,rshape[0]},module::getElementType(rhs));
loc=NameLoc::get(rewriter.getStringAttr(rname+"_to2dim"));
autorrsop=rewriter.create(loc,newType,ValueRange{rhs});
operands.push_back(rrsop);
operands.push_back(none);
//用MatMulOp實(shí)現(xiàn)[a,1]x[1,b]=[a,b],并替換原來的EinSum操作
rewriter.setInsertionPoint(op);
automatmulOp=rewriter.create(op.getLoc(),op.getType(),operands,attrs);
op.replaceAllUsesWith(matmulOp.getOperation());
rewriter.eraseOp(op);
}elseif(mode=="abcd,cde->abe"){
//可以轉(zhuǎn)換成矩陣乘法[a*b,c*d]x[c*d,e]->[a*b,e]->[a,b,e]
//lhs_reshape_rst=[lhs_shape[0]*lhs_shape[1],lhs_shape[2]*lhs_shape[3]]
rewriter.setInsertionPointAfter(lhs.getDefiningOp());
autonewType=RankedTensorType::get({lshape[0]*lshape[1],lshape[2]*lshape[3]},module::getElementType(lhs));
autoloc=NameLoc::get(rewriter.getStringAttr(lname+"_to2dim"));
autolrsOp=rewriter.create(loc,newType,ValueRange{lhs});
operands.push_back(lrsOp);
newType=RankedTensorType::get({rshape[0]*rshape[1],rshape[2]},module::getElementType(rhs));
if(module::isWeight(rhs)){
rhs.setType(newType);
operands.push_back(rhs);
}else{
rewriter.setInsertionPointAfter(rhs.getDefiningOp());
loc=NameLoc::get(rewriter.getStringAttr(rname+"_to2dim"));
autorrsop=rewriter.create(loc,newType,ValueRange{rhs});
operands.push_back(rrsop);
}
operands.push_back(none);
rewriter.setInsertionPoint(op);
newType=RankedTensorType::get({lshape[0]*lshape[1],rshape[2]},module::getElementType(op));
loc=NameLoc::get(rewriter.getStringAttr(name+"_matmul"));
automatmulOp=rewriter.create(loc,newType,operands,attrs);
autoorsOp=rewriter.create(op.getLoc(),op.getType(),ValueRange{matmulOp});
op.replaceAllUsesWith(orsOp.getOperation());
rewriter.eraseOp(op);
}elseif(mode=="abcd,bed->abce"){
rewriter.setInsertionPointAfter(rhs.getDefiningOp());
//轉(zhuǎn)換過程
//batchmatmuldoesnotsupportbroadcast
//temporarysolution
//[h,k,c]->[1,h,k,c]->[b,h,k,c]
operands.push_back(lhs);
RankedTensorTypenewType;
//右操作數(shù)處理
if(autowOp=dyn_cast(rhs.getDefiningOp())){
//對(duì)于Weight來說,可以將數(shù)據(jù)復(fù)制,解決不支持廣播問題,[b,e,d]->[a,b,e,d]
autostorage_type=module::getStorageType(rhs);
assert(storage_type.isF32()&&"Todo,supoortmoreweighttype");
autodata=wOp.read_as_byte();
uint8_t*dptr;
newType=RankedTensorType::get({lshape[0],rshape[0],rshape[1],rshape[2]},module::getElementType(rhs));
std::vector<float_t>new_filter(newType.getNumElements(),0);
dptr=(uint8_t*)new_filter.data();
//實(shí)際的數(shù)據(jù)復(fù)制過程
for(int32_ti=0;i0];i++){
autooffset=i*data->size();
memcpy(dptr+offset,data->data(),data->size());
}
autonew_op=top::create(op,"folder",new_filter,newType);
wOp.replaceAllUsesWith(new_op.getDefiningOp());
operands.push_back(new_op);
rewriter.eraseOp(wOp);
}else{
//對(duì)于普通tensor,先reshape成[1,b,e,d]再用tile算子翻倍數(shù)據(jù)為[a,b,e,d]
//Reshape操作
autoloc=NameLoc::get(rewriter.getStringAttr(rname+"_reshape"));
newType=RankedTensorType::get({1,rshape[0],rshape[1],rshape[2]},module::getElementType(rhs));
autorrsop=rewriter.create(loc,newType,ValueRange{rhs});
//Tile操作,各維tile倍數(shù)[a,1,1,1]
newType=RankedTensorType::get({lshape[0],rshape[0],rshape[1],rshape[2]},module::getElementType(rhs));
loc=NameLoc::get(rewriter.getStringAttr(rname+"_tile"));
attrs.push_back(rewriter.getNamedAttr("tile",rewriter.getI64ArrayAttr({lshape[0],1,1,1})));
autotileOp=rewriter.create(loc,newType,ValueRange{rrsop},attrs);
attrs.clear();
operands.push_back(tileOp);
}
operands.push_back(none);
//這里使用了右操作數(shù)轉(zhuǎn)置的批量矩陣乘法算子,硬件可直接支持
//[a*b,c,d]*[a*b,e,d]^T->[a*b,c,e]
attrs.push_back(rewriter.getNamedAttr("right_transpose",rewriter.getBoolAttr(true)));
rewriter.setInsertionPoint(op);
automatmulOp=rewriter.create(op.getLoc(),op.getType(),operands,attrs);
op.replaceAllUsesWith(matmulOp.getOperation());
rewriter.eraseOp(op);
}elseif(mode=="abcd,ced->abce"){
//dumbimplementation
//轉(zhuǎn)置lhs[a,b,c,d]->[a,c,b,d]
//trans_shape=[lhs_shape[0],lhs_shape[2],lhs_shape[1],lhs_shape[3]]
rewriter.setInsertionPointAfter(lhs.getDefiningOp());
autoloc=NameLoc::get(rewriter.getStringAttr(lname+"_trans"));
autonewType=RankedTensorType::get({lshape[0],lshape[2],lshape[1],lshape[3]},module::getElementType(lhs));
attrs.push_back(rewriter.getNamedAttr("order",rewriter.getI64ArrayAttr({0,2,1,3})));
autotranOp=rewriter.create(loc,newType,ValueRange{lhs},attrs);
attrs.clear();
operands.push_back(tranOp);
//復(fù)制或Tilelhs:[c,e,d]->[a,c,e,d]
rewriter.setInsertionPointAfter(rhs.getDefiningOp());
if(autowOp=dyn_cast(rhs.getDefiningOp())){
//Weight翻倍數(shù)據(jù)
autostorage_type=module::getStorageType(rhs);
assert(storage_type.isF32()&&"Todo,supoortmoreweighttype");
autodata=wOp.read_as_byte();
uint8_t*dptr;
newType=RankedTensorType::get({lshape[0],rshape[0],rshape[1],rshape[2]},module::getElementType(rhs));
std::vector<float_t>new_filter(newType.getNumElements(),0);
dptr=(uint8_t*)new_filter.data();
for(int32_ti=0;i0];i++){
autooffset=i*data->size();
memcpy(dptr+offset,data->data(),data->size());
}
autonew_op=top::create(op,"folder",new_filter,newType);
wOp.replaceAllUsesWith(new_op.getDefiningOp());
operands.push_back(new_op);
rewriter.eraseOp(wOp);
}else{
//rehshape+tile:[c,e,d]-reshape->[1,c,e,d]-tile->[a,c,e,d]
loc=NameLoc::get(rewriter.getStringAttr(rname+"_reshape"));
newType=RankedTensorType::get({1,rshape[0],rshape[1],rshape[2]},module::getElementType(rhs));
autorrsop=rewriter.create(loc,newType,ValueRange{rhs});
loc=NameLoc::get(rewriter.getStringAttr(rname+"_tile"));
attrs.push_back(rewriter.getNamedAttr("tile",rewriter.getI64ArrayAttr({lshape[0],1,1,1})));
newType=RankedTensorType::get({lshape[0],rshape[0],rshape[1],rshape[2]},module::getElementType(rhs));
autotileOp=rewriter.create(loc,newType,ValueRange{rrsop},attrs);
attrs.clear();
operands.push_back(tileOp);
}
operands.push_back(none);
//右操作數(shù)帶轉(zhuǎn)置批量矩陣乘法:[a*c, b, d]*[a*c, e, d]^T ->[a*c, b, e]->[a, c, b, e]
newType=RankedTensorType::get({lshape[0],lshape[2],lshape[1],rshape[1]},module::getElementType(op));
attrs.push_back(rewriter.getNamedAttr("right_transpose",rewriter.getBoolAttr(true)));
rewriter.setInsertionPoint(op);
loc=NameLoc::get(rewriter.getStringAttr(name+"_matmul"));
automatmulOp=rewriter.create(loc,newType,operands,attrs);
attrs.clear();
//[b,w,h,k]->[b,h,w,k]
attrs.push_back(rewriter.getNamedAttr("order",rewriter.getI64ArrayAttr({0,2,1,3})));
autotranBackOp=rewriter.create(op.getLoc(),op.getType(),ValueRange{matmulOp},attrs);
op.replaceAllUsesWith(tranBackOp.getOperation());
rewriter.eraseOp(op);
}elseif(mode=="abcd,abed->abce"||mode=="abcd,abde->abce"){
//lhs(abcd)*rhs(abed)^T->abce
//lhs(abcd)*rhs(abde)->abce
autonewType=RankedTensorType::get({lshape[0],lshape[1],lshape[2],rshape[2]},module::getElementType(op));
if(mode=="abcd,abde->abce"){
newType=RankedTensorType::get({lshape[0],lshape[1],lshape[2],rshape[3]},module::getElementType(op));
}
rewriter.setInsertionPoint(op);
rewriter.setInsertionPointAfter(rhs.getDefiningOp());
operands.push_back(lhs);
operands.push_back(rhs);
operands.push_back(none);
if(mode=="abcd,abed->abce"){
//rhs(abed)^T
attrs.push_back(rewriter.getNamedAttr("right_transpose",rewriter.getBoolAttr(true)));
}
autoloc=NameLoc::get(rewriter.getStringAttr(name));
automatmulOp=rewriter.create(loc,newType,operands,attrs);
op.replaceAllUsesWith(matmulOp.getOperation());
attrs.clear();
rewriter.eraseOp(op);
} elseif(mode=="abcd,cde->abce"){
//lhs:
//abcd->acbd(pemute)
//rhs:
//cde->1cde(reshape)
//acde->acde(tile)
//matmul:
//lhs(acbd)*rhs(acde)=result(acbe)
//result:
//acbe->abce(pemute)
//success!
rewriter.setInsertionPointAfter(lhs.getDefiningOp());
autoloc=NameLoc::get(rewriter.getStringAttr(lname+"_trans"));
autonewType=RankedTensorType::get({lshape[0],lshape[2],lshape[1],lshape[3]},module::getElementType(lhs));
attrs.push_back(rewriter.getNamedAttr("order",rewriter.getI64ArrayAttr({0,2,1,3})));
autotranOp=rewriter.create(loc,newType,ValueRange{lhs},attrs);
attrs.clear();
operands.push_back(tranOp);
rewriter.setInsertionPointAfter(rhs.getDefiningOp());
if(autowOp=dyn_cast(rhs.getDefiningOp())){
autodata=wOp.read_as_byte();
uint8_t*dptr;
newType=RankedTensorType::get({lshape[0],rshape[0],rshape[1],rshape[2]},module::getElementType(rhs));
std::vector<float_t>new_filter(newType.getNumElements(),0);
dptr=(uint8_t*)new_filter.data();
for(int32_ti=0;i0];i++){
autooffset=i*data->size();
memcpy(dptr+offset,data->data(),data->size());
}
autonew_op=top::create(op,"folder",new_filter,newType);
wOp.replaceAllUsesWith(new_op.getDefiningOp());
operands.push_back(new_op);
rewriter.eraseOp(wOp);
}else{
loc=NameLoc::get(rewriter.getStringAttr(rname+"_reshape"));
newType=RankedTensorType::get({1,rshape[0],rshape[1],rshape[2]},module::getElementType(rhs));
autorrsop=rewriter.create(loc,newType,ValueRange{rhs});
loc=NameLoc::get(rewriter.getStringAttr(rname+"_tile"));
attrs.push_back(rewriter.getNamedAttr("tile",rewriter.getI64ArrayAttr({lshape[0],1,1,1})));
newType=RankedTensorType::get({lshape[0],rshape[0],rshape[1],rshape[2]},module::getElementType(rhs));
autotileOp=rewriter.create(loc,newType,ValueRange{rrsop},attrs);
attrs.clear();
operands.push_back(tileOp);
}
operands.push_back(none);
newType=RankedTensorType::get({lshape[0],lshape[2],lshape[1],rshape[2]},module::getElementType(op));
rewriter.setInsertionPoint(op);
loc=NameLoc::get(rewriter.getStringAttr(name+"_matmul"));
automatmulOp=rewriter.create(loc,newType,operands,attrs);
attrs.clear();
attrs.push_back(rewriter.getNamedAttr("order",rewriter.getI64ArrayAttr({0,2,1,3})));
autotranBackOp=rewriter.create(op.getLoc(),op.getType(),ValueRange{matmulOp},attrs);
op.replaceAllUsesWith(tranBackOp.getOperation());
rewriter.eraseOp(op);
}else{
llvm_unreachable("Einsumnotsupportthismodenow");
}
returnsuccess();
}
總結(jié)
TPU-MLIR對(duì)EinSum的實(shí)現(xiàn)雖然不完全,但已經(jīng)足夠?qū)嵱?,能滿足目前常見網(wǎng)絡(luò)的需求。通過Converter直接表達(dá)式規(guī)范化,降低了編譯器優(yōu)化或模式分析的復(fù)雜性。在算子分析時(shí),我們不僅需要在計(jì)算上實(shí)現(xiàn)等價(jià)變換,還需充分了解實(shí)際硬件的特性。針對(duì)不同硬件架構(gòu)及其對(duì)算子的支持情況,需具體分析以找到最佳實(shí)現(xiàn)方法。此外,我們可以看到在工程實(shí)踐中,人們更注重實(shí)用性和效率,在實(shí)現(xiàn)上不必追求完備,是要覆蓋實(shí)際應(yīng)用場景即可。EinSum的轉(zhuǎn)換還有改進(jìn)空間,我們也歡迎社區(qū)提出寶貴的建議并貢獻(xiàn)代碼。
-
前端
+關(guān)注
關(guān)注
1文章
200瀏覽量
17853 -
代碼
+關(guān)注
關(guān)注
30文章
4834瀏覽量
69116 -
TPU
+關(guān)注
關(guān)注
0文章
145瀏覽量
20790
發(fā)布評(píng)論請(qǐng)先 登錄
相關(guān)推薦
Imagination D系列GPU:關(guān)于2D 雙速率紋理處理
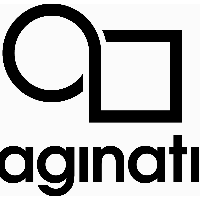
TPU編程競賽系列|第九屆集創(chuàng)賽“算能杯”火熱報(bào)名中!
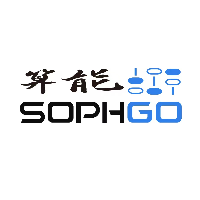
使用ADS1274 ADC進(jìn)行前端信號(hào)采集,前端信號(hào)調(diào)理過程中是否還需要設(shè)計(jì)AA Filter?
光纜用tpu外護(hù)套用在哪些型號(hào)光纜上
ADS1284 MFLG應(yīng)該怎么處理?
處理器指令的獲取過程
PLC水處理過濾器運(yùn)維管理系統(tǒng)解決方案
從TPU v1到Trillium TPU,蘋果等科技公司使用谷歌TPU進(jìn)行AI計(jì)算
PLC對(duì)模擬量信號(hào)的處理過程及方法 詳解版
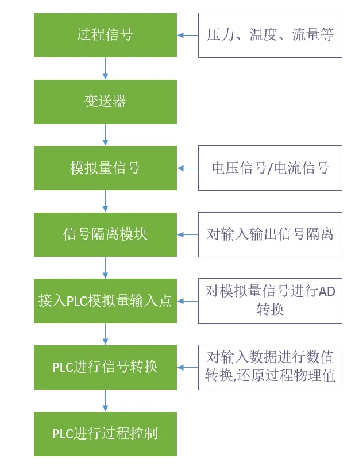
【算能RADXA微服務(wù)器試用體驗(yàn)】+ GPT語音與視覺交互:2,圖像識(shí)別
自然語言處理過程的五個(gè)層次
谷歌將推出第六代數(shù)據(jù)中心AI芯片Trillium TPU
maixcam部署yolov5s 自定義模型
大語言模型(LLMs)如何處理多語言輸入問題

評(píng)論